Product2Vec
P2V Methodology
The Product2Vec model analyzes co-occurrences of products in shopping baskets. The idea is that products that frequently appear together in customer transactions (i.e., items bought in the same shopping trip) likely share some hidden, or latent, attributes. For example, if hot dogs and hot dog buns often appear together in baskets, the model interprets them as related, even without explicit knowledge of their categories. In addition, the model understand products' similarity if the products occur in similar shopping baskets, even if they never, or very rarely, occur together (e.g., two different detergents brands). By doing this for large datasets of transactions, P2V automatically identifies relationships across a retailer’s full assortment.
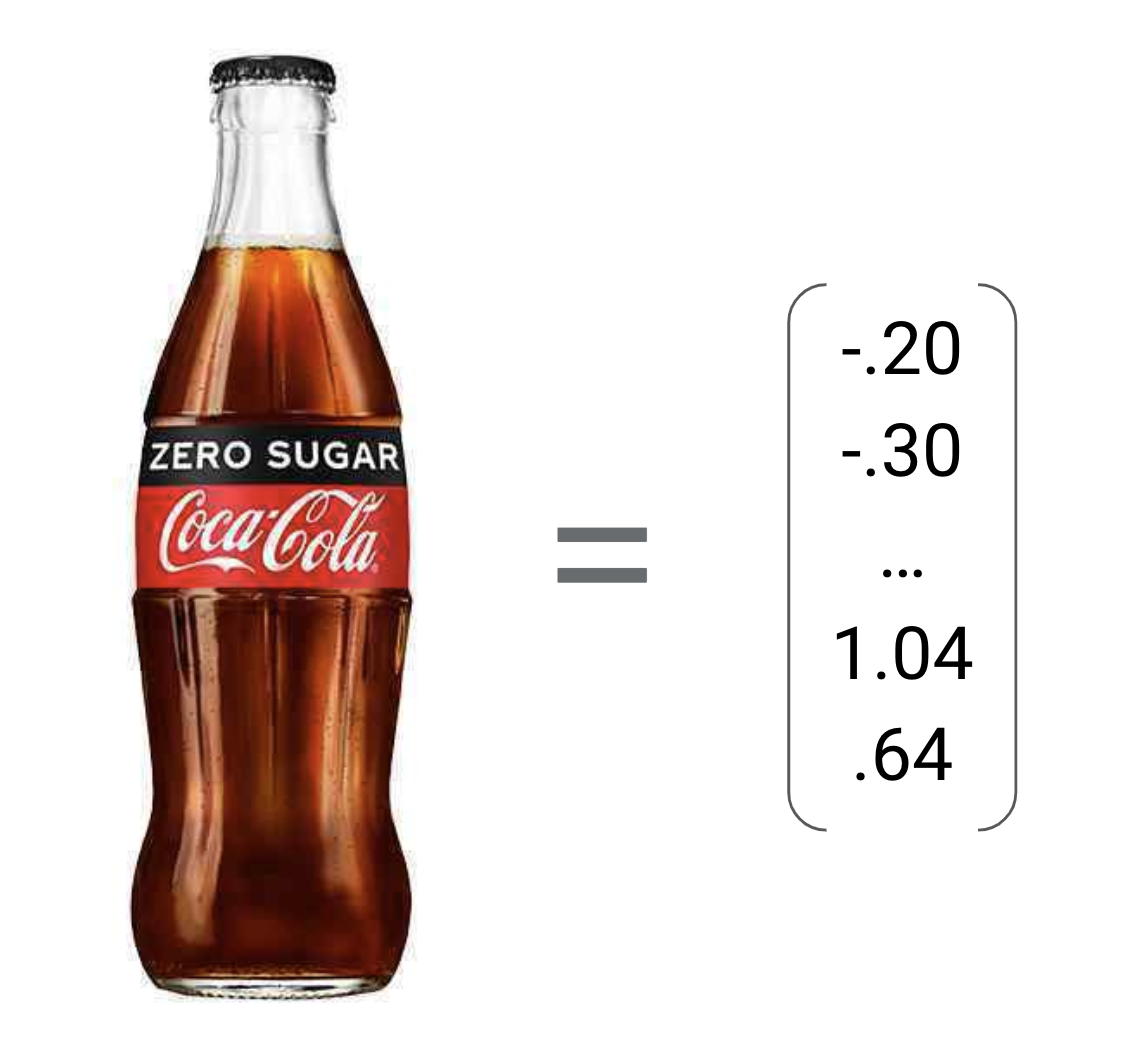
Each product is represented by D-dimensional vector. P2V generates product vectors by training a neural network model using market basket data. The training task is framed as a binary classification problem, where the model learns to predict whether two products co-occur in the same basket. For each product pair, the model produces a similarity score, and the training objective is to classify product pairs as either co-occurring (positive samples) or not co-occurring (negative samples). The loss function used is the binary cross-entropy loss, which penalizes incorrect predictions based on the dot product of the product vectors. The learning procedure involves stochastic gradient descent (SGD), where product vectors are iteratively updated to minimize the classification error. Negative sampling is employed to efficiently handle the large number of product pairs that do not co-occur, ensuring scalability to large datasets.
Fun with Product Vectors
To illustrate that product vectors capture meaningful information, consider the following
example. We take the product vector for Diet Coke
, add the product vector for Gilette
Fusion
razor blades, and subtract the product vector for Gilette Venus
razor blades.
Out of more than 30,000 product vectors, the vector most similar to Diet Coke + Gilette
Fusion - Gilette Venus
is Coke Zero
. Both difference, Coke Zero - Diet Coke
and
Gilette Fusion - Gilette Venus
capture the different in the products’ target audience.
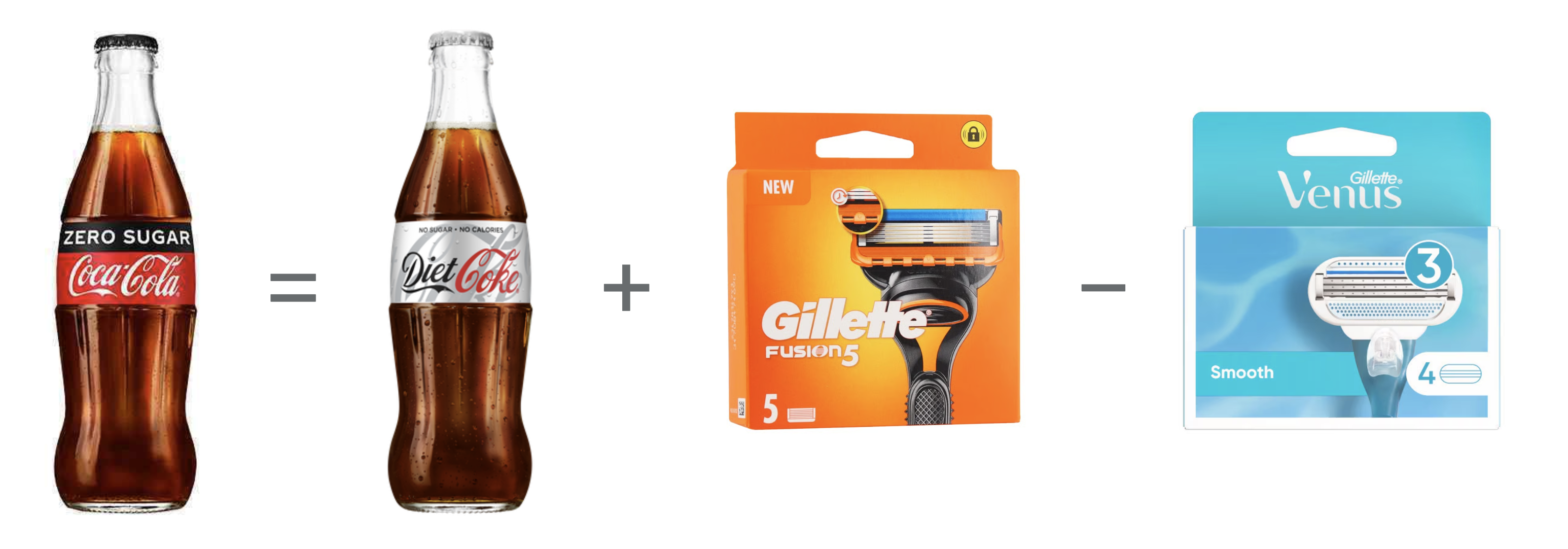
The table below illustrates that similar calculations work for a variety of other product characteristics, such as packaging size, private label, gluten-free and so on.
Attribute | Product A | Product B | Product C | Product D |
---|---|---|---|---|
Low-calorie | Fanta Orange 1l | Coca-Cola Zero 2l | Coca-Cola 2l | Fanta Orange Zero 1l |
Frozen | Mars 6pc | Snickers ice cream 6pc | Snickers 6pc | Mars ice cream 6pc |
Organic | Bananas | Onions red 500g organic | Onions red 500g | Bananas organic |
Private label | Jacobs coffee pads 16pc | PL coffee 500g | Jacobs coffee 500g | PL coffee pads 20pc |
Alcohol-free | Krombacher Pilsner .33l | Rotkäppchen .75l alcohol free | Rotkäppchen .75l | Krombacher Pilsner .33l alcohol free |
Low-fat | Geramont camembert 200g 60% fat | Bärenmarke milk 1l 1.5% fat | Bärenmarke milk 1l 3.8% fat | Geramont camembert 200g light 16% fat |
Gluten-free | Leibniz biscuits 200g | Dr. Schär Zwieback 165g | Brandt Zwieback 225g | Dr. Schär biscuits 165g |
Lactose-free | Bärenmarke whipping cream 32% | Minus L(actose) coffee creamers 10% | Bärenmarke 8% coffee creamers | Minus L(actose) whipping cream 33% |
Vegetarian | Rügenwalder bologna 80g | Mühlen meatballs 165g vegetarian | Mühlen meatballs 165g | Rügenwalder bologna 80g vegetarian |
Flavor | Landliebe yogurt 150g strawberry | Schwartau jam 340g cherry | Schwartau jam 340g strawberry | Landliebe yogurt 150g cherry |
Category | Cherry tomatoes 250g | PL carrot juice .5l | Carrots | PL tomato juice .5l JP |
Size | JP Chenet Syrah .75l | Rotkäppchen .25l | Rotkäppchen .75l | Chenet Syrah .25l |
Multi-pack | Hohes C multivitamins 1l | Hohes C orange 3x.2l | Hohes C orange 1l | Hohes C multivitamins 3x.2l |
Packaging | Italian parsley 50g bunch | French parsley 40g flowpack | French parsley 50g bunch | Italian parsley 40g flowpack |
Season | Lindt Easter bunny 100g | Merci Santa Claus 120g | Merci Easter bunny 120g | Lindt Santa Claus 70g |